I’ve gone on about natural philosophy, the philosophy of representation, science history, and the importance of interdisciplinary perspectives when studying modern science. There’s something that unifies all these ideas, and I wouldn’t have thought of it at all hadn’t I spoken to the renowned physicist Dr. George Sterman on January 3.
I was attending the Institute of Mathematical Sciences’ golden jubilee celebrations. A lot of my heroes were there, and believe me when I say my heroes are different from your heroes. I look up to people who are capable of thinking metaphysically, and physicists more than anyone I’ve come to meet are very insightful in that area.
One such physicist is Dr. Ashoke Sen, whose contributions to the controversial area of string theory are nothing short of seminal – if only for how differently it says we can think about our universe and what the math of that would look like. Especially, Sen’s research into tachyon condensation and the phases of string theory is something I’ve been interested in for a while now.
Knowing that George Sterman was around came as a pleasant surprise. Sterman was Sen’s doctoral guide; while Sen’s a string theorist now, his doctoral thesis was in quantum chromodynamics, a field in which the name of Sterman is quite well-known.
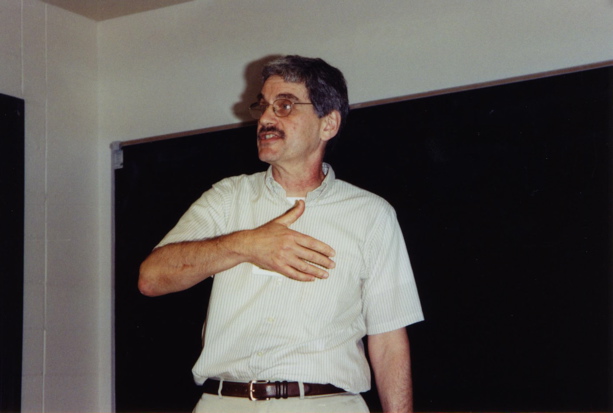
– DR. GEORGE STERMAN (IMAGE: UC DAVIS)
When I finally got a chance to speak with Sterman, it was about 5 pm and there were a lot of mosquitoes around. We sat down in the middle of the lawn on a couple of old chairs, and with a perpetual smile on his face that made one of the greatest thinkers of our time look like a kid in a candy store, Sterman jumped right into answering my first question on what he felt about the discovery of a Higgs-like boson.
Where Sheldon Stone was obstinately practical, Sterman was courageously aesthetic. After the (now usual) bit about how the discovery of the boson was a tribute to mathematics and its ability to defy 50 years of staggering theoretical advancements by remaining so consistent, he said, “But let’s think about naturalness for a second…”
The moment he said “naturalness”, I knew what he was getting it, but more than anything else, I was glad. Here was a physicist who was still looking at things aesthetically, especially in an era where lack of money and the loss of practicality by extension could really put the brakes on scientific discovery. I mean it’s easy to jump up and down and be excited about having spotted the Higgs, but there are very few who feel free to still not be happy.
In Sterman’s words, uttered while waving his arms about to swat away the swarming mosquitoes while discussing supersymmetry:
“There’s a reason why so many people felt so confident about supersymmetry. It wasn’t just that it’s a beautiful theory – which it is – or that it engages and challenges the most mathematically oriented among physicists, but in another sense in which it appeared to be necessary. There’s this subtle concept that goes by the name of naturalness. Naturalness as it appears in the Standard Model says that if we gave our any reasonable estimate of what the mass of the Higgs particle should be, it should by all rights be huge! It should be as heavy as what we call the Planck mass [~10^19 GeV].”
Or, as Martinus Veltman put it in an interview to Matthew Chalmers for Nature,
“Since the energy of the Higgs is distributed all over the universe, it should contribute to the curvature of space; if you do the calculation, the universe would have to curve to the size of a football.”
Naturalness is the idea in particle physics specifically, and in nature generally, that things don’t desire to stand out in any way unless something’s really messed up. For instance, consider the mass hierarchy problem in physics: Why is the gravitational force so much more weaker than the electroweak force? If either of them is a fundamental force of nature, then where is the massive imbalance coming from?
Formulaically speaking, naturalness is represented by this equation:
Here, lambda (the mountain) is the cut-off scale, an energy scale at which the theory breaks down. Its influence over the naturalness of an entity h is determined by how many dimensions lambda acts on – with a maximum of 4. Last, c is the helpful scaling constant that keeps lambda from being too weak or too strong in some setting.
In other words, a natural constant h must be comparable to other nature constants like it if they’re all acting in the same setting.
(TeX: hquad =quad c{ Lambda }^{ 4quad -quad d })
However, given how the electroweak and gravitational forces – which do act in the same setting (also known as our universe) – differ so tremendously in strength, the values of these constants are, to put it bluntly, coincidental.
Problems such as this “violate” naturalness in a way that defies the phenomenological aesthetic of physics. Yes, I’m aware this sounds like hot air but bear with me. In a universe that contains one stupendously weak force and one stupendously strong force, one theory that’s capable of describing both forces would possess two disturbing characteristics:
1. It would be capable of angering one William of Ockham
2. It would require a dirty trick called fine-tuning
I’ll let you tackle the theories of that William of Ockham and go right on to fine-tuning. In an episode of ‘The Big Bang Theory’, Dr. Sheldon Cooper drinks coffee for what seems like the first time in his life and goes berserk. One of the things he touches upon in a caffeine-induced rant is a notion related to the anthropic principle.
The anthropic principle states that it’s not odd that the value of the fundamental constants seem to engender the evolution of life and physical consciousness because if those values aren’t what they are, then a consciousness wouldn’t be able to observe them. Starting with the development of the Standard Model of particle physics in the 1960s, it’s become known that these constants are really fine in their value.
So, with the anthropic principle providing a philosophical cushioning, like some intellectual fodder to fall back on when thoughts run low, physicists set about trying to find out why the values are what they are. As the Standard Model predicted more particles – with annoying precision – physicists also realised that given the physical environment, the universe would’ve been drastically different even if the values were slightly off.
Now, as discoveries poured in and it became clear that the universe housed two drastically different forces in terms of their strength, researchers felt the need to fine-tune the values of the constants to fit experimental observations. This sometimes necessitated tweaking the constants in such a way that they’d support the coexistence of the gravitational and electroweak forces!
Scientifically speaking, this just sounds pragmatic. But just think aesthetically and you start to see why this practice smells bad: The universe is explicable only if you make extremely small changes to certain numbers, changes you wouldn’t have made if the universe wasn’t concealing something about why there was one malnourished kid and one obese kid.
Doesn’t the asymmetry bother you?
Put another way, as physicist Paul Davies did,
“There is now broad agreement among physicists and cosmologists that the Universe is in several respects ‘fine-tuned’ for life. The conclusion is not so much that the Universe is fine-tuned for life; rather it is fine-tuned for the building blocks and environments that life requires.”
(On a lighter note: If the universe includes both a plausible anthropic principle and a Paul Davies who is a physicist and is right, then multiple universes are a possibility. I’ll let you work this one out.)
Compare all of this to the desirable idea of naturalness and what Sterman was getting at and you’d see that the world around us isn’t natural in any sense. It’s made up of particles whose properties we’re sure of, of objects whose behaviour we’re sure of, but also of forces whose origins indicate an amount of unnaturalness… as if something outside this universe poked a finger in, stirred up the particulate pea-soup, and left before anyone evolved enough to get a look.
(This blog post first appeared at The Copernican on January 6, 2013.)